-
Notifications
You must be signed in to change notification settings - Fork 21.4k
New issue
Have a question about this project? Sign up for a free GitHub account to open an issue and contact its maintainers and the community.
By clicking “Sign up for GitHub”, you agree to our terms of service and privacy statement. We’ll occasionally send you account related emails.
Already on GitHub? Sign in to your account
Bilinear interpolation behavior inconsistent with TF, CoreML and Caffe #10604
Comments
Thanks for the report. We will look into this! |
I've run a few experiments and it seems that Pytorch with |
For pytorch implementation, here's the logic to compute src_idx <-> dst_idx mapping. |
So do you think there is any workaround in Pytorch to make it's interpolation consistent with that of TensorFlow? |
Using half_pixel_centers = True along with align_corners = False in tf.image.resize_bilinear will work same as torch.nn.functional.interpolate with align_corners = False |
This is a PyTorch implementation of import torch
import numpy as np
def tf_consistent_bilinear_upsample(imgs, scale_factor=1.0):
b, c, h, w = imgs.shape
assert h == w
N = int(h * scale_factor)
delta = (1.0 / h)
p = int(scale_factor) - 1
xs = torch.linspace(-1.0 + delta, 1.0 - delta, N - p)
ys = torch.linspace(-1.0 + delta, 1.0 - delta, N - p)
grid = torch.meshgrid(xs, ys)
gridy = grid[1]
gridx = grid[0]
gridx = torch.nn.functional.pad(gridx.unsqueeze(0), (0, p, 0, p), mode='replicate')[0]
gridy = torch.nn.functional.pad(gridy.unsqueeze(0), (0, p, 0, p), mode='replicate')[0]
grid = torch.stack([gridy, gridx], dim=-1).unsqueeze(0).repeat(b, 1, 1, 1)
output = torch.nn.functional.grid_sample(imgs, grid, mode='bilinear', padding_mode='zeros')
return output The code is tested and the results are consistent with Tensorflow when the scale factor is an integer value. |
This is the correct solution, I tested the result on 1 dimension, the loss is less than 0.01. |
Issue description
Trying to compare and transfer models between Caffe, TF and Pytorch found difference in output of bilinear interpolations between all. Caffe is using depthwise transposed convolutions instead of straightforward resize, so it's easy to reimplement both in TF and Pytorch.
However, there is difference between output for TF and Pytorch with
align_corners=False
, which is default for both.Code example
But
Output diff * 10:
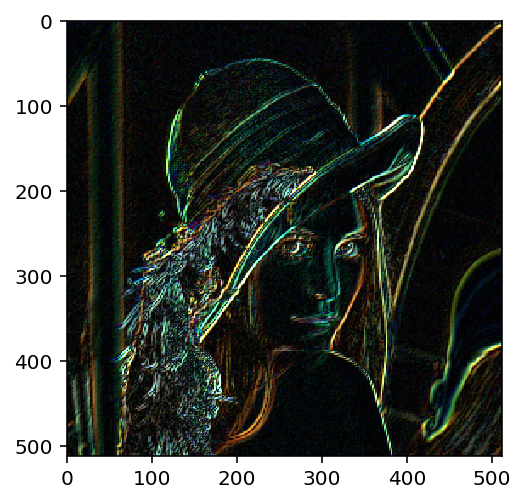
Output of CoreML is consistent with TF, so it seems that there is a bug with implementation of bilinear interpolation with
align_corners=False
in Pytorch.Diff is reproducible both on cpu and cuda with cudnn 7.1, cuda 9.1.
The text was updated successfully, but these errors were encountered: