You signed in with another tab or window. Reload to refresh your session.You signed out in another tab or window. Reload to refresh your session.You switched accounts on another tab or window. Reload to refresh your session.Dismiss alert
I want to use ResNet50 as my model and change Fully Connection layer to classify images.
#Create model:
model = models.resnet50(pretrained=True)
fc_inputs = model.fc.in_features
class FClayer(nn.Module):
def init(self):
super(FClayer, self).init()
self.fc1 = nn.Linear(fc_inputs, 2)
self.relu1 = nn.ReLU()
self.fc2 = nn.Linear(2, num_classes)
def forward(self, x):
x = self.relu1(self.fc1(x))
y = self.fc2(x)
return x, y
model.fc = FClayer()
...
#In train function:
features, outputs = model(inputs)
alpha = 1
loss = loss_criterion(outputs, labels) + (loss_criterion_cent(features, labels) * alpha)
optimizer.zero_grad()
optimizer_cent.zero_grad()
loss.backward()
optimizer.step()
for param in loss_criterion_cent.parameters():
param.grad.data *= (1./alpha)
optimizer_cent.step()
I dont know why when i was training, at the very first epochs, the accuracy had increased, but soon later, nothing happended. Here is my accuracy plot curve chart:
Do I have something wrong?
The text was updated successfully, but these errors were encountered:
Your question is quite ambiguous. But I think it was probably caused by a high LR or a high loss weight which makes gradient descent optimization failed.
I want to use ResNet50 as my model and change Fully Connection layer to classify images.
#Create model:
model = models.resnet50(pretrained=True)
fc_inputs = model.fc.in_features
class FClayer(nn.Module):
def init(self):
super(FClayer, self).init()
self.fc1 = nn.Linear(fc_inputs, 2)
self.relu1 = nn.ReLU()
self.fc2 = nn.Linear(2, num_classes)
def forward(self, x):
x = self.relu1(self.fc1(x))
y = self.fc2(x)
return x, y
model.fc = FClayer()
...
#In train function:
features, outputs = model(inputs)
alpha = 1
loss = loss_criterion(outputs, labels) + (loss_criterion_cent(features, labels) * alpha)
optimizer.zero_grad()
optimizer_cent.zero_grad()
loss.backward()
optimizer.step()
for param in loss_criterion_cent.parameters():
param.grad.data *= (1./alpha)
optimizer_cent.step()
I dont know why when i was training, at the very first epochs, the accuracy had increased, but soon later, nothing happended. Here is my accuracy plot curve chart:
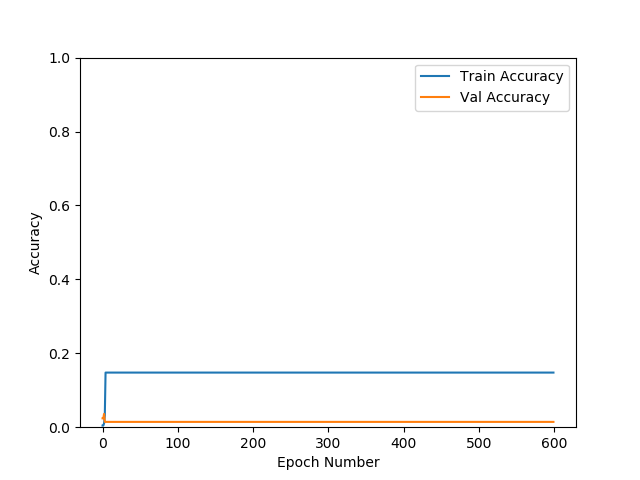
Do I have something wrong?
The text was updated successfully, but these errors were encountered: